CMPlaces is designed to train and evaluate cross-modal scene recognition models. It covers five different modalities: natural images, sketches, clip-art, text descriptions, and spatial text images. Each example in the dataset is annotated with one of the 205 scene labels from Places, which is one of the largest scene datasets available today. Hence, the examples in our dataset span a large number of natural situations. Examples in our dataset are not paired between modalities, encouraging researches to develop methods that learn strong alignments from weakly aligned data.
We chose these modalities for two reasons. Firstly, since the goal of the dataset is to study transfer across significantly different modalities, we seek modalities with different statistics to those of natural images (such as line drawings and text). Secondly, these modalities are easier to generate than real images, which is relevant to applications such as image retrieval. In total, Cross-Modal Places contains more than ~1 million images comprising 205 unique scene categories and 5 modalities.
Please cite one of the following papers if you use this service:
Learning Aligned Cross-Modal Representations from Weakly Aligned Data
Ll. Castrejón*, Y. Aytar*, C. Vondrick, H. Pirsiavash and A. TorralbaCVPR 2016
Cross-Modal Scene Networks
Y. Aytar*, Ll. Castrejón*, C. Vondrick, H. Pirsiavash and A. TorralbaIn Submission
Notice: Please do not overload our server by querying repeatedly in a short period of time. This is a free service for academic research and education purposes only. It has no guarantee of any kind. For any questions or comments regarding this demo, please contact the authors.
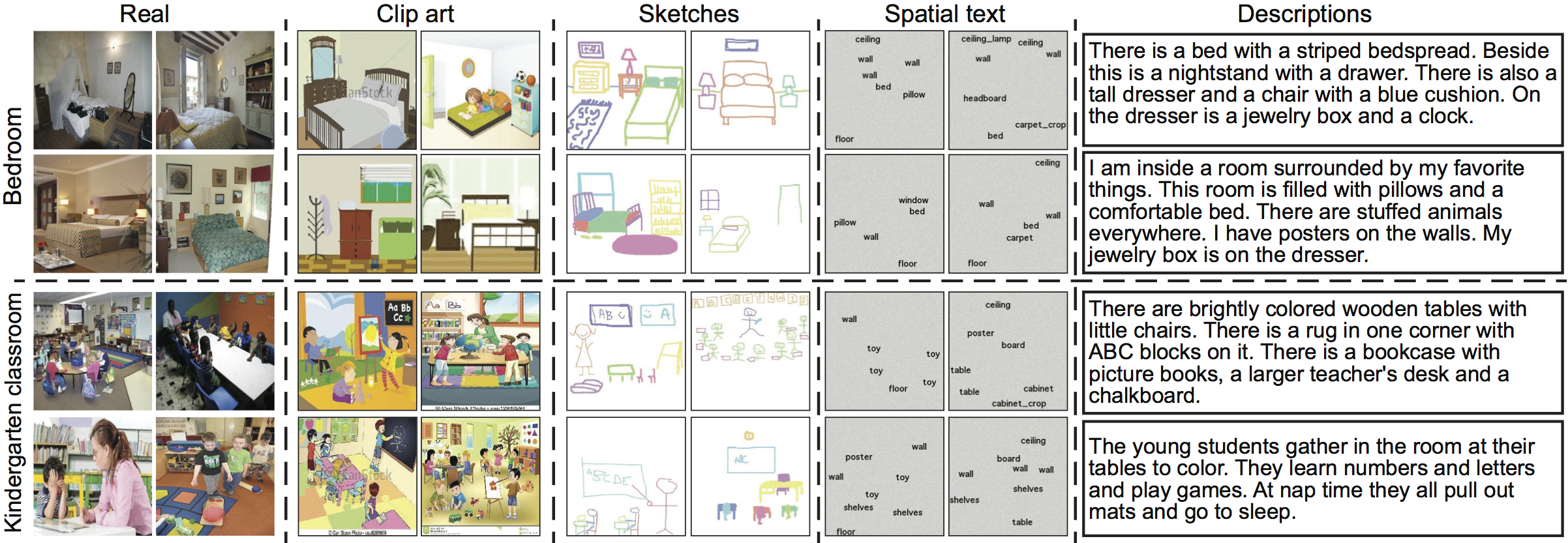
We thank TIG for managing our computer cluster. We gratefully acknowledge the support of NVIDIA Corporation with the donation of the GPUs used for this research. This work was supported by NSF grant IIS-1524817, by a Google faculty research award to A.T and by a Google Ph.D. fellowship to C.V.